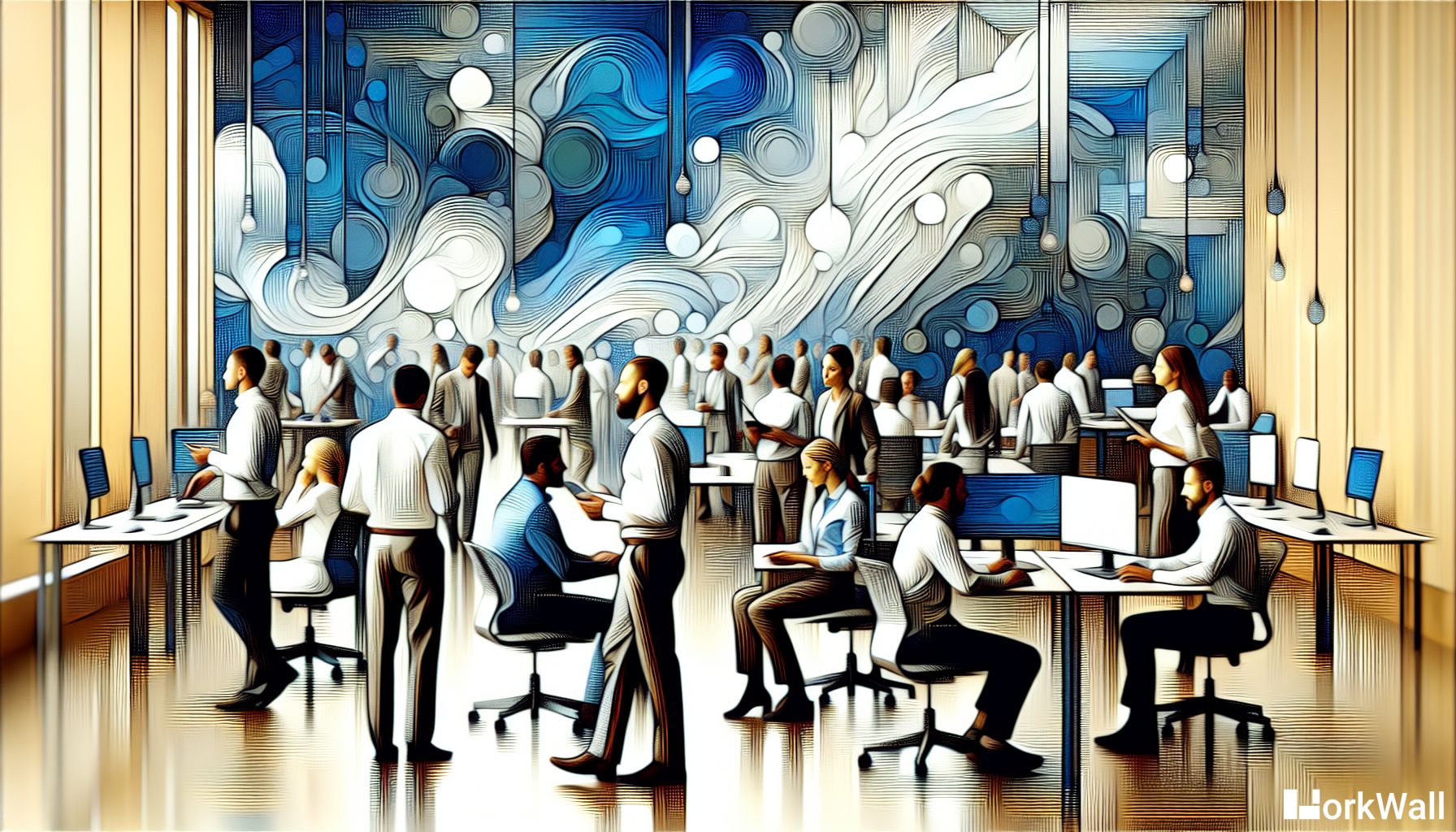
Leveraging TensorFlow for AI-Driven Business Analytics

.png)
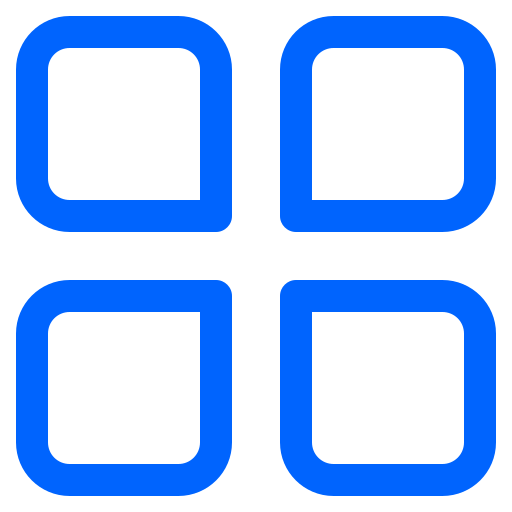
Unveiling the Power of TensorFlow in Business Analytics
Welcome to a deep dive into the revolutionary realm of TensorFlow and its applications in business analytics! Whether you are a seasoned data scientist, a curious functional user, or an end user interested in the latest tech trends, this detailed exploration is tailored just for you. Our journey will encompass practical applications, scalability, and integration techniques, transforming raw data into strategic business insights. So, gear up as we unfold how TensorFlow, along with platforms like Workwall, is pioneering data-driven decision-making processes.
Understanding TensorFlow
TensorFlow, developed by the engineers and researchers of the Google Brain team, is an open-source software library designed for high-performance numerical computation. It’s extensively used to develop Machine Learning (ML) models and deep learning neural networks. With its flexible, yet powerful ecosystem, TensorFlow not only helps in research and development of advanced ML models but also provides robust tools to deploy these models in production environments.
Key Features:
- Flexibility and Modularity: TensorFlow allows you to assemble graphs of data flow and functions that align with the unique needs of your enterprise without compromising on scalability and performance.
- Massive Collaboration: Being open-source means there’s a vast community out there to support, contribute and collaborate, enhancing the tool continuously.
- Portability: TensorFlow models can run on multiple CPUs or GPUs, and on desktop, server, or mobile platforms which makes it ideal for businesses that operate on diverse platforms.
From Data to Insights: Practical Applications of TensorFlow in Business
TensorFlow excels in crunching huge datasets and extracting meaningful insights. Let's break down some real-world scenarios where TensorFlow is currently being leveraged:
1. Customer Behavior Prediction
Companies across various sectors are using TensorFlow to predict customer behaviors like buying patterns and product preferences. This information is crucial for improving product recommendations and personalizing experiences which directly influence sales performance.
2. Risk Management
In the financial sector, TensorFlow models help predict loan default risks, thereby assisting banks in making informed decisions. The proactive identification of risks contributes to better portfolio management and reduced financial losses.
3. Efficient Resource Management
Manufacturing and logistics companies implement TensorFlow algorithms to optimize their supply chains and production lines. Predictive maintenance of machinery, improved stock management, and enhanced delivery schedules are only a few of the benefits.
4. Enhanced Medical Diagnoses
Healthcare providers leverage TensorFlow to facilitate faster and more accurate diagnoses. AI models can predict patient deterioration and assist in early detection of diseases which significantly improves patient outcomes and operational efficiencies.
Scalability: Growing with TensorFlow
Focusing on scalability, TensorFlow supports distributed computing, allowing you to expand computation across multiple data sets and GPUs. This is particularly beneficial for businesses as it means they can scale their operations without significant delays or disruptions.
Utilize platforms like Workwall that facilitate marketplace dynamics, ensuring your scalable TensorFlow models integrate seamlessly with existing systems, thus boosting analytics capabilities without reinventing the wheel.
Integration Techniques for TensorFlow Models
Effective integration of TensorFlow into your business processes is pivotal. Here’s how you can ensure smooth integration:
- Data Preparation: Standardize and preprocess your data to improve the efficacy of your TensorFlow models. Garbage in, garbage out!
- Model Training: Utilize TensorFlow’s extensive libraries and community support to train the model with your preprocessed data.
- Deployment: Deploy the trained model into your production environment. Tools like TensorFlow Serving or TensorFlow Lite can help depending on your application requirement.
- Monitoring and Optimization: Continuously monitor the model's performance and tweak it based on feedback and data changes. Regular updates will help in maintaining its accuracy and relevance.
Conclusion: Jump on the TensorFlow Bandwagon!
Implementing TensorFlow for business analytics doesn't have to be daunting. With a clear strategy for application, scalability consideration, and thoughtful integration, businesses can unleash the power of their data. Remember, every large dataset has a treasure trove of insights waiting to be unlocked, and TensorFlow is your key!
Stay tuned to our blog that we'll keep spiced up with marketplace trends, industry humor, and invaluable insights. Don't forget to check out Workwall for synergizing with market dynamics and accelerating your analytics journey. Ready to transform data into actionable business strategies with TensorFlow? Let’s get rolling!
And hey, if you enjoyed reading this, why not share it with your colleagues or on your social media platforms? Let's spread the knowledge and together, let's scale new heights in analytics!
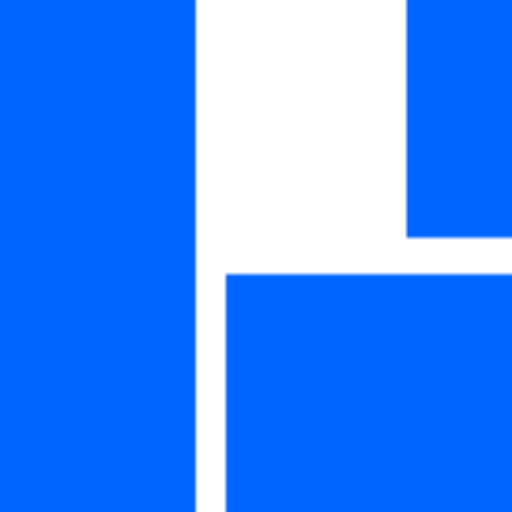
Follow us
Interested in more content like this? Follow us on